On January 15, the Dutch government was forced to resign amidst a scandal around its child-care benefits scheme. Systems that were meant to detect misuse of the benefits scheme, mistakenly labelled over 20,000 parents as fraudsters. More crucially, a disproportionate amount of those labelled as fraudsters had an immigration background.
Amongst the upheaval, little attention was brought to the fact that the tax authority was making use of algorithms to guide its decision-making. In a report by the Dutch Data Protection Authority, it became clear that a ‘self-learning’ algorithm was used to classify the benefit claims. Its role was to learn which claims had the highest risk of being false. The risk-classification model served as a first filter; officials then scrutinized the claims with the highest risk label. As it turns out, certain claims by parents with double citizenship were systematically identified by the algorithm as high-risk, and officials then hastily marked those claims as fraudulent.
It is difficult to identify what led the algorithm to such a biased output, and that is precisely one of the core problems. This blogpost argues that the Dutch scandal should serve as a cautionary lesson for agencies who want to make use of algorithmic enforcement tools and stresses the need for dedicated governance structures within such agencies to prevent missteps.
The problem of fairness in machine learning
The Dutch scandal places us at the centre of the crucial debate around algorithmic fairness (Kleinberg et al., 2018). As this case shows, mistakes in the implementation of machine learning, can distort reality and lead to unfair outcomes. Such mistakes, like the so-called ‘class imbalance’, a common machine learning classification problem (Buda et al., 2018), may have been a factor which led the authority’s algorithm to find a greater distribution of errors in a minority population. The output of the algorithm consequently became biased and unfair.
We argue that this concept can easily be extended beyond this specific case. Algorithmic bias within agencies could adversely affect any stakeholder in any sector. Depending on various aspects such as the nature of the data that it processes (Lum, 2017), certain stakeholders could find themselves adversely labelled, while others are not. The algorithm of a competition agency, for instance, could disproportionally focus on firms in a particular region. Or food and safety controls could be unfairly directed to a specific type of food.
A tempting tool for supervisors
As the EU moves to more risk-based enforcement strategies (Blanc & Faure, 2020), it may be appealing for agencies to use algorithms to assess risk. This is particularly the case in sectors where the caseload would benefit from some form of automated data analysis. Though machine readable data is a prerequisite for such tools, most agencies have an abundance of it. The ECB, for instance, has expressed its interest in machine learning techniques to make supervision more efficient and proactive. It already attempted using algorithms to simplify fit and proper assessments of bank board appointees. Despite the appeal, the Dutch welfare scandal has highlighted the risks of using them in enforcement and the need for a dedicated governance structure.
The need for a dedicated governance structure
Recently, the European Commission focussed its AI concerns on the protection of fundamental rights and values. It also set out Ethics guidelines for trustworthy AI, in which fairness (or the absence of bias) is one of the key requirements of AI systems. Bias is described as ‘an inclination of prejudice towards or against a person, object, or position’. Fairness should therefore ensure that a risk-based model does not affect smaller groups of stakeholders disproportionately.
Beyond the broad strokes of principled commitments, agencies will need to devise meticulous plans to assess the operations of its machine learning algorithms. Removing bias from algorithm is an extremely difficult task (Hao, 2019) and requires true expertise. As the EBA notes, bias prevention and detection is a continuously evolving field of research. Ideally, one should ensure that algorithms are free of bias by design. This can sometimes only be achieved by hypothesising about the algorithm’s potential effects (Corbett-Davies & Goel, 2018). Agencies will therefore need to continuously adjust their algorithms, a task which may require dedicated departments and expertise.
Agencies may be inclined to hide behind the fact that, ultimately, competent humans control the input and output of the algorithm. But it is precisely because humans control the input and output of algorithms, that the mistakes they may make could ultimately transpire in the algorithm. The Dutch algorithm was ultimately trained by tax officials who, consciously or not, may have ‘taught’ the algorithm the bias they already had. Agencies therefore cannot escape the due diligence simply because the algorithm is merely used as a support tool.
Furthermore, the more complex the rules are, the more their enforcement requires flexibility. The rigidity of an algorithm may be useful as a classifier but will not grasp all the nuances of individual situations. There is a real risk of new types of false positives and false negatives. An algorithm may correctly estimate the risk in a very specific scenario, but this should not dictate enforcement policy.
Agencies may be more vulnerable
The ECB recently stated that : “supervisors are still responsible for their work, even if part of it is performed by a computer”. While this seems like a reasonable postulate, it overlooks the fact that the consequences of algorithmic enforcement are not limited to the responsibility of supervisors. It is likely that the more enforcement relies on algorithms, the more exposed it becomes to the risks associated with it.
From an agency design perspective, algorithmic enforcement may significantly reduce the accountability of agencies if management boards do not equip themselves with the necessary expertise. The deference to an algorithm may weaken the agency’s internal accountability, by attributing decisions to a less explainable machine. On the long run this could affect the effectiveness of enforcement.
The Dutch government could find solace in its political resignation, agencies are in a more delicate situation. Those which heavily rely on legitimacy to foster compliance (Tyler, 2003), could be the ones most hardly struck by an algorithmic mistake through undermining of their credibility. Such mistakes could also shatter strong deterrence-based enforcement strategies, which rely on the ability to identify risk accurately.
Legitimacy also comes paired with transparency, agencies must be clear about when they use algorithms and how they use them. Such transparency however comes with a risk. As stakeholders innovate to lower the cost of the regulatory burden, the most resourceful amongst them may exploit the functioning of enforcement algorithms to remain under the radar.
A cautionary tale
The Dutch benefits scandal teaches us a lesson about the risks of algorithmic enforcement. The difference lies in the fact that the tax authority had the luxury of political accountability, allowing it to mitigate long-term consequences of the scandal. An algorithmic scandal in an agency may be more prone to do lasting damage to its legitimacy, and thus, the effectiveness of its enforcement. Agencies should therefore put in place dedicated governance structures to ensure algorithms are free from bias and are used responsibly. A recently leaked version of the Commission’s draft AI regulation, expected to also apply to EU agencies (article 2(2)(d)), may be a good first step to guide them.
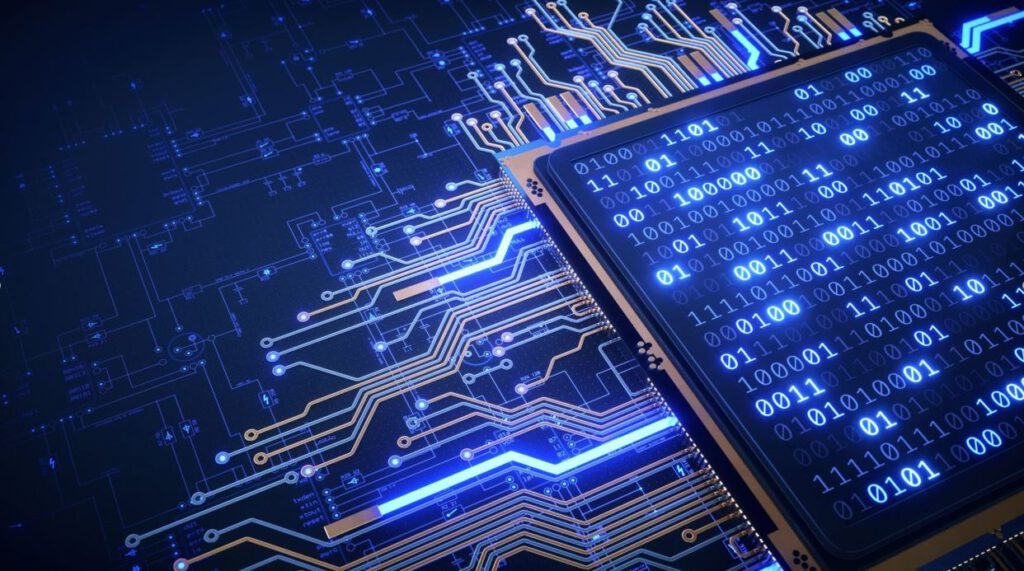
A small correction. You write that:
‘As it turns out, certain claims by parents with double citizenship were systematically identified by the algorithm as high-risk, and officials then hastily marked those claims as fraudulent.’
This is not correct. The selection algorithm did not assign a higher score to persons with dual nationality where one of the nationalities was Dutch. Rather, the system ‘penalised’ (in the sense of: assigning a higher risk score) persons who (only) had a foreign nationality. See the DPA report, page. 43.